Prof. Ben Y. Zhao to give keynote talk at The 5th International Workshop on Complex Networks and their Applications
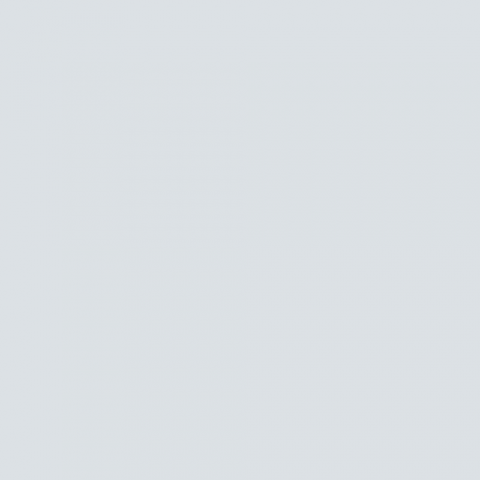
Title
An Empirical View of Link Prediction in Social Networks
Abstract
Algorithms based on complex networks affect our online experience on a daily basis. One of the most ubiquitous examples of this is the link prediction problem, which is a core part of friend recommendations on social networks like LinkedIn, Facebook, and Pinterest, and also part of broader recommendation systems like personal live streaming on Periscope or Q&A sites like Quora. Given the success of these systems, and the decade of work on link prediction, it is reasonable to assume that this is a solved problem. Yet no quantitative study has been performed to understand just how successful (or unsuccessful) these algorithms are. Meanwhile, there are plenty of anecdotes online of poor recommendations that represent poor prediction results (e.g. Kashmir Hill, Fusion 2016). In this talk, I will present some of our recent work on taking an empirical view to the well-studied problem of link prediction in dynamic networks. We implement and apply 18 link prediction algorithms (some metric-based, some machine learning based) to several traces of detailed network dynamics (Renren, Facebook, YouTube), and evaluate their prediction accuracy. We find that on absolute terms, link prediction accuracy is embarrassingly poor across the board, highlighting the fact that this is still very much an open problem. Machine learning approaches tend to outperform relatively, but are often prohibitively high computation costs. We then propose a novel approach to build "prediction filters” using past patterns in network dynamics. Evaluated on our large datasets, our results significantly boost prediction accuracy across all algorithms.