CS Colloquium: Yuheng Bu
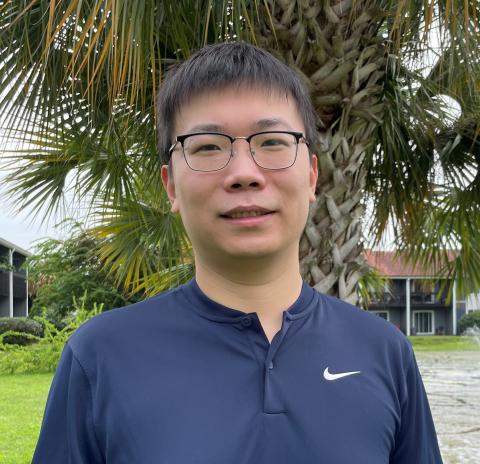
Speaker: Yuheng Bu
Date: Monday, October 3, 2022
Time: 3:30 - 4:30 pm
Location: HFH 1132
Host: Shiyu Chang
Title: Can Information Theory Characterize Learning Algorithms?
Abstract:
Information theory has guided the practical communication system design by characterizing the fundamental limits of data communication and compression. This talk will discuss how methodologies originating from information theory can provide similar benefits in learning problems. We show that information-theoretic tools can be used to understand the generalization behavior of learning algorithms, i.e., how a trained machine learning model behaves on unseen data. We provide an exact characterization of the generalization error for the Gibbs algorithm, which can be viewed as a randomized empirical risk minimization algorithm. We show that the generalization error of the Gibbs algorithm is equal to the symmetrized Kullback-Leibler (KL) information between the input training samples and the output model weights. Such an information-theoretic approach is versatile, as we can also characterize the generalization error of some transfer learning algorithms and improve model compression algorithms. We believe this analysis can guide the choice of transfer learning algorithms and the design of the learning system in practice.
Bio:
Dr. Yuheng Bu is an Assistant Professor in the Department of Electrical & Computer Engineering (ECE) at the University of Florida. Before joining the University of Florida, he was a postdoctoral research associate at the Research Laboratory of Electronics and Institute for Data, Systems, and Society (IDSS), Massachusetts Institute of Technology (MIT). He received a B.S. degree (Hons.) in EE from Tsinghua University in 2014 and a Ph.D. degree in electrical and computer engineering from the University of Illinois at Urbana Champaign in 2019. His research interests lie at the intersection of machine learning, signal processing, and information theory.