UCSB Computer Science makes strong showing at NeurIPS 2023
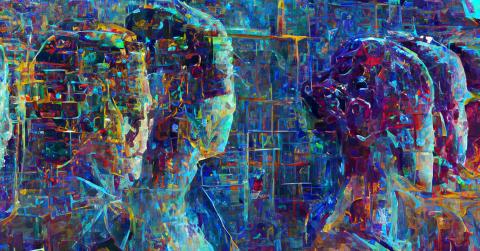
UC Santa Barbara Computer Science is set to make a strong showing at this year’s Neural Information Processing Systems (NeurIPS) annual meeting, with 20 papers accepted at the prestigious conference. With contributions from professors William Wang, Yu-Xiang Wang, Shiyu Chang, and Michael Beyeler on topics as wide-ranging as text-to-image evaluation, privacy, and predictive models of visual cortex neural activity, UCSB’s efforts mirror the broad scope of the conference itself.
Intended to foster the exchange of machine learning research in relation to various biological, technological, mathematical, and theoretical fields, NeurIPS is an important hub for interdisciplinary thought. With invited lecturers, workshops, and oral and poster presentations of refereed papers, 2023 marks the conference’s 37th year of bringing together researchers in machine learning, neuroscience, computer vision, natural language processing, life and social sciences, and adjacent fields.
“NeurIPS is an influential event in the areas of AI and ML,” says Divy Agrawal, chair of the Computer Science Department at UCSB. “Having a single publication in this conference is considered a great achievement for a research group. Publication of tens of papers in NeurIPS from UCSB signifies the influential research being conducted at UCSB in the areas of AI/ML. I should also mention that the NeurIPS 2023 success is consistent with the UC Santa Barbara spirit of having large research impact with a relatively small research footprint.”
In addition to the many papers headed to the conference this year from UCSB contributors, Associate Professor Yu-Xiang Wang has two spotlight presentations: “Online Label Shift: Optimal Dynamic Regret Meets Practical Algorithms”; and “A Privacy-Friendly Approach to Data Visualization.”
A few other highlights from UCSB contributors:
- William Wang’s team will unveil LLMScore, a new evaluation framework for large language model (LLM) text-to-image synthesis; LayoutGPT for text-to-image compositional visual planning with human-level performance in designing numerical and spatially correct layouts; and Multimodal C4, an open, billion-scale corpus of images interleaved with text. Additionally, they will show how LLMs can be viewed as topic models, improving in-context learning by 12.5% through optimized demonstration selection.
- Yu-Xiang Wang‘s team will show their focus on privacy and reinforcement learning in the previously mentioned spotlight presentations, but also in papers such as “Improving the Privacy and Practicality of Objective Perturbation for Differentially Private Linear Learners”; “Offline Reinforcement Learning with Differential Privacy”; and “Automatic Clipping: Differentially Private Deep Learning Made Easier and Stronger.”
- Shiyu Chang and his team will address dataset pruning for transfer learning in computer vision tasks. Their proposal offers two pretraining pruning methods applicable to a range of paradigms, resulting in reduction of source data classes by 40 to 80% with no loss of downstream performance, along with increases of two to five times in the speed of model pretraining.
- Michael Beyeler’s lab will show the results of their explorations into biologically constrained deep learning architectures, demonstrating how insights from neuroscience can enrich current deep learning architectures. They’ve also examined human-in-the-loop optimization for deep stimulus encoding in visual prostheses, along with another study of a multimodal recurrent neural network investigating V1 activity and behavioral and temporal dynamics in freely moving mice.
NeurIPS 2023 will draw researchers from around the world to New Orleans, Louisiana, from December 10 to 16. Previous meetings were held in locations as varied as Montréal, Lake Tahoe, and Barcelona, Spain.