Prof. Ben Zhao gives keynote at IEEE IPCCC
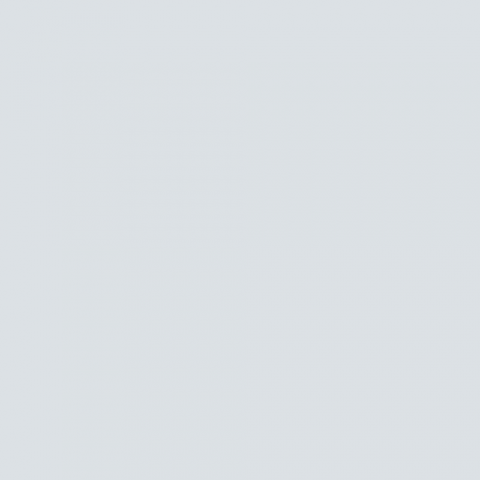
Professor Ben Zhao delivered a keynote talk on December 1, 2012 at the IEEE International Performance Computing and Communications Conference in Austin, TX. His talk was entitled “A Double-edged Sword: Implications of Crowdsourcing for the Future of Web Security.”
Abstract Despite recent advancements in the areas of machine learning and data mining, there are still areas where human effort cannot be easily replicated by software systems. The rise of crowdsourcing systems such as Amazon Turk help fills this void by enabling the mobilization of human effort for large-scale computing tasks. Like many new techniques or systems, crowdsourcing systems are a double-edged sword capable of being used for either “good” or “evil.” In this talk, I will take a closer look at the impact of crowdsourcing on the security of web-services. I will focus on both positive and negative implications of crowdsourcing systems for the future. First, I will discuss how crowdsourcing can help us address difficult problems in dealing with fake online identities in online social networks. I will describe recent work studying crowdsourced Sybil detection, using a large user study and several ground-truth datasets of fake and real users. Results show that in the right conditions, human workers can be highly accurate in identifying real and fake identities. In fact, we can build scalable systems for crowdsourced Sybil detection, and data from user studies show that it can provide highly accurate results with very low cost. Second, I will discuss the negative implications of crowdsourcing, and describe a detailed measurement study on malicious “crowdturfing” systems, where users sign up to perform tasks such as spreading rumors, writing fake reviews, and creating fake online identities. Through both large scale data measurements and experiments, our study shows that these systems are highly scalable, and growing exponentially in both jobs and revenue, and pose a new type of threat to the security of today’s online communities. Abstract: Despite recent advancements in the areas of machine learning and data mining, there are still areas where human effort cannot be easily replicated by software systems. The rise of crowdsourcing systems such as Amazon Turk help fills this void by enabling the mobilization of human effort for large-scale computing tasks. Like many new techniques or systems, crowdsourcing systems are a double-edged sword capable of being used for either “good” or “evil.” In this talk, I will take a closer look at the impact of crowdsourcing on the security of web-services. I will focus on both positive and negative implications of crowdsourcing systems for the future. First, I will discuss how crowdsourcing can help us address difficult problems in dealing with fake online identities in online social networks. I will describe recent work studying crowdsourced Sybil detection, using a large user study and several ground-truth datasets of fake and real users. Results show that in the right conditions, human workers can be highly accurate in identifying real and fake identities. In fact, we can build scalable systems for crowdsourced Sybil detection, and data from user studies show that it can provide highly accurate results with very low cost. Second, I will discuss the negative implications of crowdsourcing, and describe a detailed measurement study on malicious “crowdturfing” systems, where users sign up to perform tasks such as spreading rumors, writing fake reviews, and creating fake online identities. Through both large scale data measurements and experiments, our study shows that these systems are highly scalable, and growing exponentially in both jobs and revenue, and pose a new type of threat to the security of today’s online communities.