CS Colloquium: Hrushikesh Mhaskar
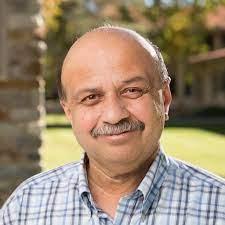
Speaker: Hrushikesh Mhaskar
Date: Monday, April 24th, 2023
Time: 3:30 - 4:30 pm
Location: HFH 1132
Host: Yu-Xiang Wang
Title: Revisiting the Theory of Machine Learning
Abstract:
The fundamental problem of machine learning is often formulated as the problem of function approximation. For example, we have data of the form {(xj , yj )}, where yj is the class label for xj , and we want to approximate the class label as a function of the input x. The standard way for this approximation is to minimize a loss functional, usually with some regularization. Surprisingly, even though the problem is posed as a problem of function approximation, approximation theory has played only a marginal role in this theory. We describe our efforts to explore why this might be the case, and also to develop approximation theory/harmonic analysis tools more meaningfully and directly applicable to machine learning.
Bio:
Hrushikesh Mhaskar (b. 1956, Pune, India) did his undergraduate studies in the Institute of Science, Nagpur, and received his first M. Sc. in mathematics from the Indian Institute of Technology in Mumbai in 1976. He received his Ph. D. in mathematics and M. S. in computer science from the Ohio State University, Columbus, in 1980. He then joined Cal. State L. A. and was promoted to full professor in 1990. After retirement in 2012, he was a visiting associate with California Institute of Technology until 2017, and occasionally served as a consultant for Qualcomm.
He has been a Research Professor at Claremont Graduate University since 2012. He has authored more than 150 articles in the area of approximation theory, potential theory, neural networks, wavelet analysis, and data processing. His book,“Weighted polynomial approximation”, was published in 1997 by World Scientific, and the book with Dr. D. V. Pai, “Fundamentals of Approximation Theory” was published by Narosa Publishers, CRC, and Alpha Science in 2000. He serves on the editorial boards of Applied and Computational Harmonic Analysis, Frontier Journal on mathematics of computation and data science, Journal of Approximation Theory, Mathematical Foundations of Computing, and Jaen Journal of Approximation. He was awarded the Humboldt Fellowship for research in Germany four times. He was the John von Neumann distinguished professor in 2011, and will be the August-Wilhelm Scheer Professor in 2020, at Technical University of Munich. His research was supported by the Intelligence Advanced Research Project Agency, the National Science Foundation, the U. S. Army Research Office, the Air Force Office of Scientific Research, the National Security Agency, and the Research and Development Laboratories.